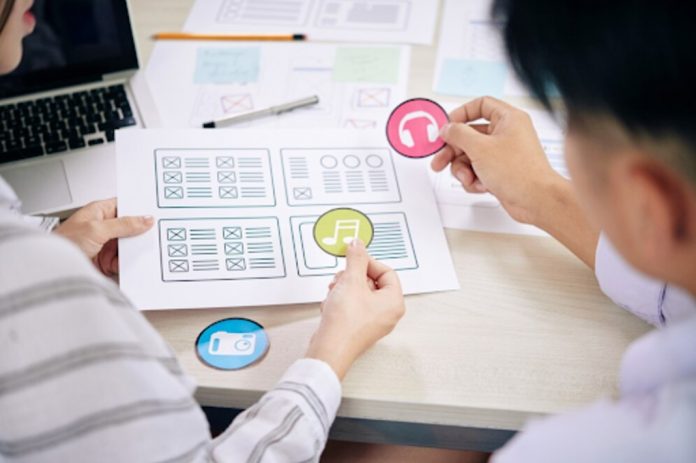
In Six Sigma, hypothesis testing is where data meets decision-making, offering a structured approach to understanding and improving processes. It’s not just a statistical exercise. It’s a critical tool that empowers businesses to challenge assumptions, validate improvements, and make evidence-based decisions. Think about it: what if you could confidently identify whether a change in your process leads to actual improvement or if it’s just a fluke? Hypothesis testing helps cut through uncertainty by providing clear, measurable answers.
From analyzing production defects to enhancing customer satisfaction, this method plays a vital role in achieving Six Sigma’s goal of near-perfect processes. By transforming raw data into actionable insights, hypothesis testing equips professionals with the confidence to back their decisions with solid evidence. In this blog, we’ll take you through the hypothesis testing process, its importance in Six Sigma, and how it drives impactful, data-driven changes that stand the test of time.
Table Of Contents
- What is Hypothesis Testing in Lean Six Sigma?
- The Hypothesis Testing Process in Six Sigma Projects
- Why is Hypothesis Testing Important for Six Sigma Projects?
- Limitations of Hypothesis Testing in Six Sigma Projects
- Conclusion
What is Hypothesis Testing in Lean Six Sigma?
Hypothesis testing in Lean Six Sigma is a statistical method used to make informed decisions by analyzing data and testing assumptions. It helps determine whether a change or difference in a process is significant or simply due to random chance. This process is essential for validating improvements and ensuring they lead to measurable, sustainable benefits.
In Lean Six Sigma, hypothesis testing is often used during the Analyze phase of the DMAIC methodology. It allows practitioners to compare two sets of data, for example, the performance of a process before and after an improvement, and confirm if the changes have had a meaningful impact.
The process typically involves two hypotheses:
- Null Hypothesis (H0): Assumes no significant difference or effect exists. For example, a process change has not improved efficiency
- Alternative Hypothesis (H1): Assumes a significant difference or effect exists. For example, the process change has improved efficiency
The Hypothesis Testing Process in Six Sigma Projects
The Six Sigma hypothesis testing process follows a structured approach to determine whether a proposed change or improvement in a process has a statistically significant effect. This process ensures that decisions made in Lean Six Sigma projects are data-driven and not based on assumptions or anecdotal evidence. Here’s a breakdown of the steps involved:
1. Define the Hypothesis
The first step in the Lean Six Sigma DMAIC cycle is to define the problem. This involves thoroughly understanding the process, identifying the specific issues, and determining the scope of the problem. Teams set measurable, clear goals that align with business objectives to ensure focused improvement efforts. This step is essential for guiding the project and setting a foundation for effective problem-solving and measurable results.
2. Select the Appropriate Test
Choose the right statistical test based on the type of data you have and the hypothesis you want to test. Common statistical tests used in Six Sigma include:
- T-test: Used when comparing the means of two groups (e.g., before and after a process change).
- ANOVA (Analysis of Variance): Used when comparing means of more than two groups.
- Chi-Square Test: Used for categorical data to see if distributions of categorical variables differ from expected outcomes.
3. Collect and Analyze Data
Gather the data needed to test your hypothesis. This can include process performance data before and after the change, defect rates, cycle times, etc. Ensure that the data is representative and sufficient to draw meaningful conclusions. Once the data is collected, it will be analyzed using the chosen statistical test.
4. Set the Significance Level (α)
The significance level (denoted as α ) is the probability of rejecting the null hypothesis when it is true. A common significance level used in Six Sigma is 0.05 (5%), which means there is a 5% chance of incorrectly rejecting the null hypothesis. This value helps determine whether the results are statistically significant.
5. Perform the Test and Calculate the p-value
Using the data, perform the statistical test and calculate the p-value, which tells you the probability of observing the data if the null hypothesis is true.
- If p-value <= α (significance level): Reject the null hypothesis (H0) and accept the alternative hypothesis (H1). This means the improvement is statistically significant.
- If p-value > α : Do not reject the null hypothesis. This suggests that the improvement is not statistically significant, and further analysis or adjustments may be needed.
6. Draw Conclusions
Based on the results of the hypothesis test, make a decision about whether the process change had a significant effect. If the null hypothesis is rejected, you can confidently say that the change led to an improvement. If the null hypothesis is not rejected, it means the change did not produce a significant improvement, and you may need to revisit the changes made or look for other factors affecting the process.
7. Take Action Based on Findings
After interpreting the results, take appropriate action. If the hypothesis test shows a significant improvement, the process change can be standardized, and the control phase can begin to maintain the improvement. If no significant change is found, you may need to investigate further, adjust your approach, or test different hypotheses.
Why is Hypothesis Testing Important for Six Sigma Projects?
Six Sigma Hypothesis testing is crucial for projects because it provides a structured, data-driven way to make decisions and validate improvements. In Six Sigma, the goal is to reduce defects and improve processes to achieve near-perfect performance. Hypothesis testing is important in ensuring that the changes made during a project are not just random occurrences but genuine improvements that can be consistently replicated.
Here’s why hypothesis testing is so important for Six Sigma projects:
1. Data-Driven Decisions
Six Sigma emphasizes making decisions based on data rather than intuition or assumptions. Hypothesis testing allows teams to analyze data and test whether the improvements made to a process are statistically significant, ensuring that changes are effective and not due to chance.
2. Validating Improvements
After making process changes, it’s essential to verify whether those changes led to real improvements. Hypothesis testing compares the results before and after the change, helping to confirm that the improvements are not just temporary or random but sustained and meaningful.
3. Minimizing Risks
In a Six Sigma project, you’re often working with critical processes. Hypothesis testing helps reduce the risk of making changes that might not lead to the expected outcomes. By testing hypotheses, teams can avoid costly mistakes and ensure that their actions will lead to measurable improvements.
4. Objective Analysis
Hypothesis testing eliminates biases and subjective judgment from the decision-making process. It provides an objective way to assess whether a proposed change or solution is effective, which is essential for maintaining credibility and consistency in Six Sigma projects.
5. Continuous Improvement
Six Sigma projects are focused on ongoing improvements. Hypothesis testing helps identify areas for further optimization by revealing whether changes lead to sustained process improvements or if additional adjustments are needed. It encourages a culture of continuous refinement based on data and evidence.
6. Proven Results
The ultimate goal of any Six Sigma project is to achieve high-quality results. Hypothesis testing provides statistical evidence to support claims of success, ensuring that the process improvements are both reliable and repeatable, driving consistent, high-quality outcomes over time.
Limitations of Hypothesis Testing in Six Sigma Projects
Hypothesis testing is a fundamental statistical tool used in Six Sigma projects to validate assumptions, compare different groups, or determine the effectiveness of improvements. However, while it is valuable, it has certain limitations that can affect the outcomes and interpretations of Six Sigma projects.
Here are the key limitations:
- Dependence on Data Quality: Hypothesis testing relies on accurate, reliable data. If the data is flawed or incomplete, the results of the hypothesis test may be misleading. Poor data collection, sampling errors, or biases can lead to incorrect conclusions
- Assumption of Normal Distribution: Many hypothesis tests assume that the data follows a normal distribution. If the data is not normally distributed and this assumption is not checked or addressed, the results may not be valid. In such cases, non-parametric tests or data transformation methods might be needed
- Sample Size Sensitivity: The power of a hypothesis test depends on the sample size. With small sample sizes, the test may not be sensitive enough to detect a significant effect, even if one exists. Conversely, with large sample sizes, even trivial differences might be deemed statistically significant, leading to false positives
- Does Not Prove Causality: Hypothesis testing can show whether a relationship or difference exists, but it cannot prove causality. In Six Sigma projects, while hypothesis testing can demonstrate that a change in a process correlates with improved performance, it does not prove that the change caused the improvement. Other external factors could be at play
- Risk of Type I and Type II Errors: Hypothesis testing carries the risk of Type I errors (false positives), where a hypothesis is incorrectly rejected, and Type II errors (false negatives), where a true effect is missed. Balancing the significance level (α) and power of the test is crucial but not always easy
- Limited Scope: Hypothesis testing typically tests a specific hypothesis at a time and may not fully capture the complexity of real-world processes. In complex systems, other variables and relationships not considered in the test also play a significant role
- Requires Expertise: Proper interpretation of hypothesis test results requires statistical knowledge. Without a solid understanding of the test’s assumptions, limitations, and appropriate use cases, there’s a risk of misinterpreting the results, which can lead to incorrect decisions
Conclusion
Hypothesis testing is an essential tool in the Six Sigma methodology, particularly during the Analyze phase of the DMAIC cycle. It enables teams to make data-driven decisions by determining whether observed changes in a process are statistically significant or simply due to chance. By carefully defining hypotheses, selecting appropriate statistical tests, and interpreting results with caution, Six Sigma practitioners can validate improvements and ensure that decisions are based on reliable evidence. While hypothesis testing is invaluable in driving process improvement, it’s important to recognize its limitations, such as reliance on data quality, sample size, and assumptions. When used correctly, hypothesis testing helps organizations optimize processes, reduce variation, and achieve measurable, sustainable results.
Ready to enhance your skills in Six Sigma and drive process improvements in your organization? Enroll in Invensis Learning’s Six Sigma and Quality Management certification courses today and gain the knowledge.